2.3 Comparisons between AI and humans
2.3.1 Breast cancer detection
In a Google Health project 8 the following results were achieved:
- Absolute reduction of 5.7% and 1.2% (USA and UK) in false positives
- Absolute reduction 9.4% and 2.7% (USA and UK)in false negatives.
In an independent study of six radiologists, the AI system outperformed all of the human readers. More on the study at https://www.nature.com/articles/s41586-019-1799-6
2.3.2 Working together: Lung cancer detection
With an estimated 160,000 deaths in 2018, lung cancer is the most common cause of cancer death in the United States
A study published in Nature medicine 9 a team of members of Google AI and several hospitals reported
When prior computed tomography imaging was not available
- Model outperformed all six radiologists
- Absolute reductions of 11% in false positives
- Absolute reductions 5% in false negatives
2.3.3 ImageNet Large Scale Visual Recognition Challenge (ILSVRC)
The ImageNet Large Scale Visual Recognition Challenge 10 (ILSVRC) (Russakovsky et al. 2015) evaluates algorithms for object recognition and image classification on a large scale.
Facts of ImageNet 11:
- 14 million images
- 20,000 image categories
- 1000 image categories used for ILSVRC
The development of the results is shown in the graph below. The number of layers is a indication of model complexity
In 2017 the problem set to status “solved”
- 29 of 38 competing teams had an accuracy of more than 95%
- ImageNet stopped competition
2.3.4 AlphaGo Zero
Go is a strategy game invented 2500 years ago and has an estimated number of possible board configuration of 10¹⁷⁴ compared to chess which has is 10¹²º. A detailed description is given by DeepMind’s blog post “AlphaGo Zero: Starting from scratch” 12
AlphaGo Zero is a version of DeepMind’s13 Go software AlphaGo
No human intervention
- No usage of historical data
- After 3 days of training as good as AlphaGo which beat world champion in 4 out of 5
- After 40 days of training becomes best Go player in the world
AlphaZero learned three games,
The capability progress of Alpha Zero during training is shown below
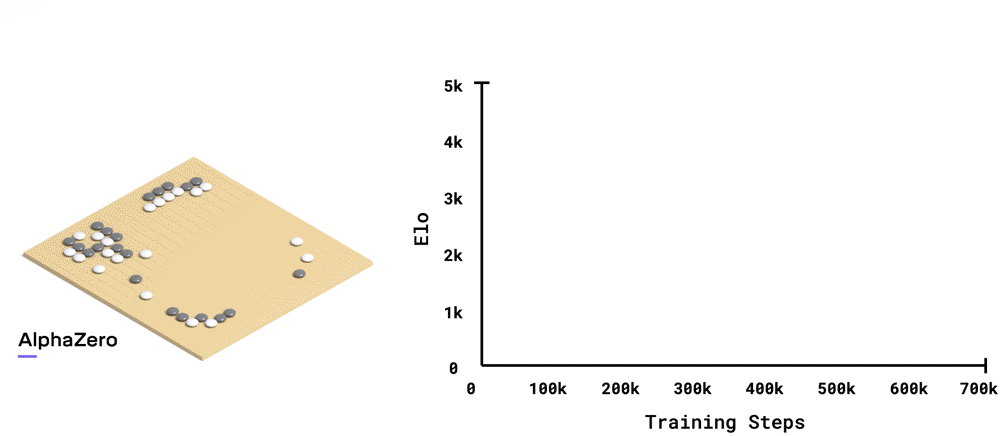
Figure from https://deepmind.com/blog/article/alphazero-shedding-new-light-grand-games-chess-shogi-and-go
NOTE: EACH TRAINING STEP REPRESENTS 4,096 BOARD POSITIONS.
At the end of the training Alpha Zero achieved the following performance:
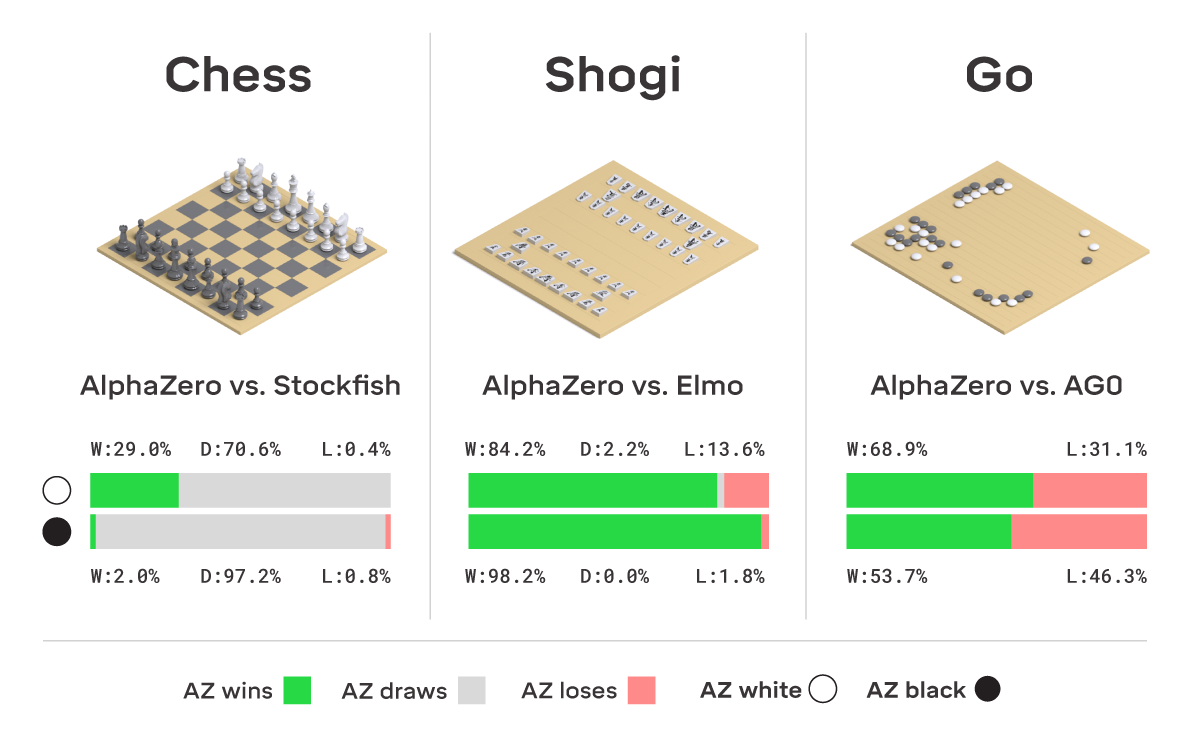
Figure from https://deepmind.com/blog/article/alphazero-shedding-new-light-grand-games-chess-shogi-and-go
Implications are wider than just playing a game, as Garry Kasparov, a former world chess champion puts it:
The implications go far beyond my beloved chessboard… Not only do these self-taught expert machines perform incredibly well, but we can actually learn from the new knowledge they produce.”
References
Screening mammography aims to identify breast cancer at earlier stages of the disease, when treatment can be more successful. Despite the existence of screening programmes worldwide, the interpretation of mammograms is affected by high rates of false positives and false negatives. Here we present an artificial intelligence (AI) system that is capable of surpassing human experts in breast cancer prediction. To assess its performance in the clinical setting, we curated a large representative dataset from the UK and a large enriched dataset from the USA. We show an absolute reduction of 5.7% and 1.2% (USA and UK) in false positives and 9.4% and 2.7% in false negatives. We provide evidence of the ability of the system to generalize from the UK to the USA. In an independent study of six radiologists, the AI system outperformed all of the human readers: the area under the receiver operating characteristic curve (AUC-ROC) for the AI system was greater than the AUC-ROC for the average radiologist by an absolute margin of 11.5%. We ran a simulation in which the AI system participated in the double-reading process that is used in the UK, and found that the AI system maintained non-inferior performance and reduced the workload of the second reader by 88%. This robust assessment of the AI system paves the way for clinical trials to improve the accuracy and efficiency of breast cancer screening. https://www.nature.com/articles/s41586-019-1799-6↩︎
Abstract With an estimated 160,000 deaths in 2018, lung cancer is the most common cause of cancer death in the United States1. Lung cancer screening using low-dose computed tomography has been shown to reduce mortality by 20–43% and is now included in US screening guidelines. Existing challenges include inter-grader variability and high false-positive and false-negative rates. We propose a deep learning algorithm that uses a patient’s current and prior computed tomography volumes to predict the risk of lung cancer. Our model achieves a state-of-the-art performance (94.4% area under the curve) on 6,716 National Lung Cancer Screening Trial cases, and performs similarly on an independent clinical validation set of 1,139 cases. We conducted two reader studies. When prior computed tomography imaging was not available, our model outperformed all six radiologists with absolute reductions of 11% in false positives and 5% in false negatives. Where prior computed tomography imaging was available, the model performance was on-par with the same radiologists. This creates an opportunity to optimize the screening process via computer assistance and automation. While the vast majority of patients remain unscreened, we show the potential for deep learning models to increase the accuracy, consistency and adoption of lung cancer screening worldwide. Website: https://www.nature.com/articles/s41591-019-0447-x↩︎
Homepage of ILSVRC http://www.image-net.org/challenges/LSVRC/↩︎
Homepage of ImageNet http://www.image-net.org↩︎
https://deepmind.com/blog/article/alphago-zero-starting-scratch↩︎
Homepage of DeepMind https://deepmind.com↩︎